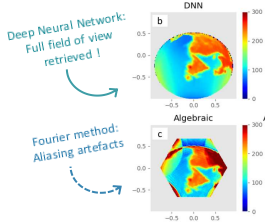
Antenna arrays such as SMOS use a technique known as aperture synthesis to increase the spatial resolution of the brightness temperature maps. Traditional imaging methods for aperture synthesis interferometers are based in Fourier theory.
In the framework of a R&T CNES project, we have proposed an alternative data-driven approach that retrieves the brightness temperature maps with noticeably less alias contamination from highly undersampled Fourier data, and thus provides a significantly wider exploitable field of view.
A representative example of reconstruction (below) demonstrates the ability of the proposed deep neural network (DNN) to reconstruct brightness temperature maps over the entirety of the field of view, from highly undersampled visibilities, with little impact of the aliasing artifacts. The resulting exploitable area comprises 2.5 times more pixels than the Earth-alias-free field of view (EAFFOV) of the algebraic approach.
The proposed DNN presents better reconstruction performances and an error distribution less spread out than the Fourier algebraic method. However, land/ocean transitions are still error prone, especially on the periphery of the reconstructed image. Ocean areas are well reconstructed anywhere.
Richard Faucheron, Eric Anterrieu, Louise Yu, Nemesio Rodriguez-Fernandez