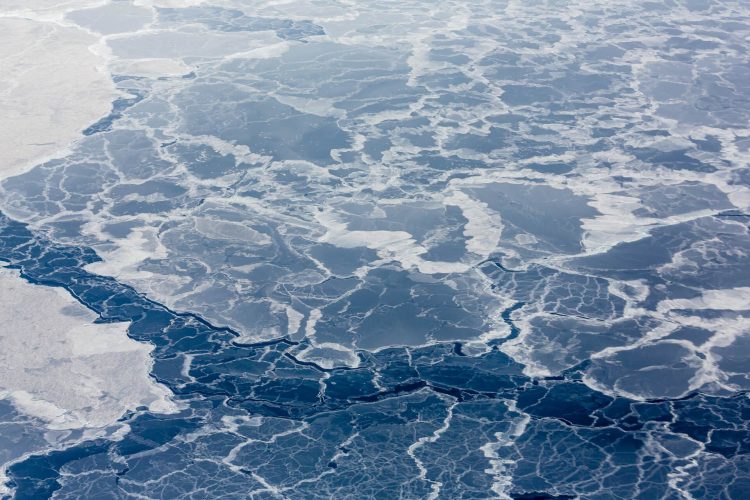
Thin sea ice is an essential component of the Arctic’s physical environment, playing a crucial role in climate and weather regulation by mediating heat and water exchange between the ocean and atmosphere. It also serves as a key indicator of climate change, and conditions ship operations in the area. SMOS – with the surface brightness temperature – can provide estimates of thin ice thickness up to 1 meter.
Currently, two algorithms are used to retrieve thin sea ice thickness from SMOS measurements: ESA’s official product, generated with a semi-empirical algorithm developed by the Alfred Wegener Institute (AWI), and an empirical approach distributed by the University of Bremen (UB).
isardSAT – an Earth Observation SME based in Barcelona, informed us of a recent study conducted with the Institut de Ciències del Mar. It presents two machine learning algorithms (Random Forest and Gradient Boosting) which, trained with SMOS brightness temperature, improves sea ice thickness estimates.
Arctic sea ice thickness map generated with the Random Forest and Gradient Boosting algorithms for 30th January 2019. © isardSAT
Indeed, when validated against ground-truth data from mooring buoys of the Beaufort Gyre Exploration Project (BGEP), the results show an even higher correlation compared to the existing ESA product, moderately reducing some of its errors.
Buoy from the Beaufort Gyre Exploration Project (BGEP) measuring sea ice thickness. © Beaufort Gyre Exploration Project (BGEP)
“In the future, advanced deep learning algorithms like these should be explored to improve not only spatial but also temporary consistency of satellite data”, explains Ferran Hernández Macià, researcher involved in the project. “This could help to obtain reliable near-real time sea ice predictions, necessary for ship operations, but also to study the consequences of the climate change”, adds the researcher.