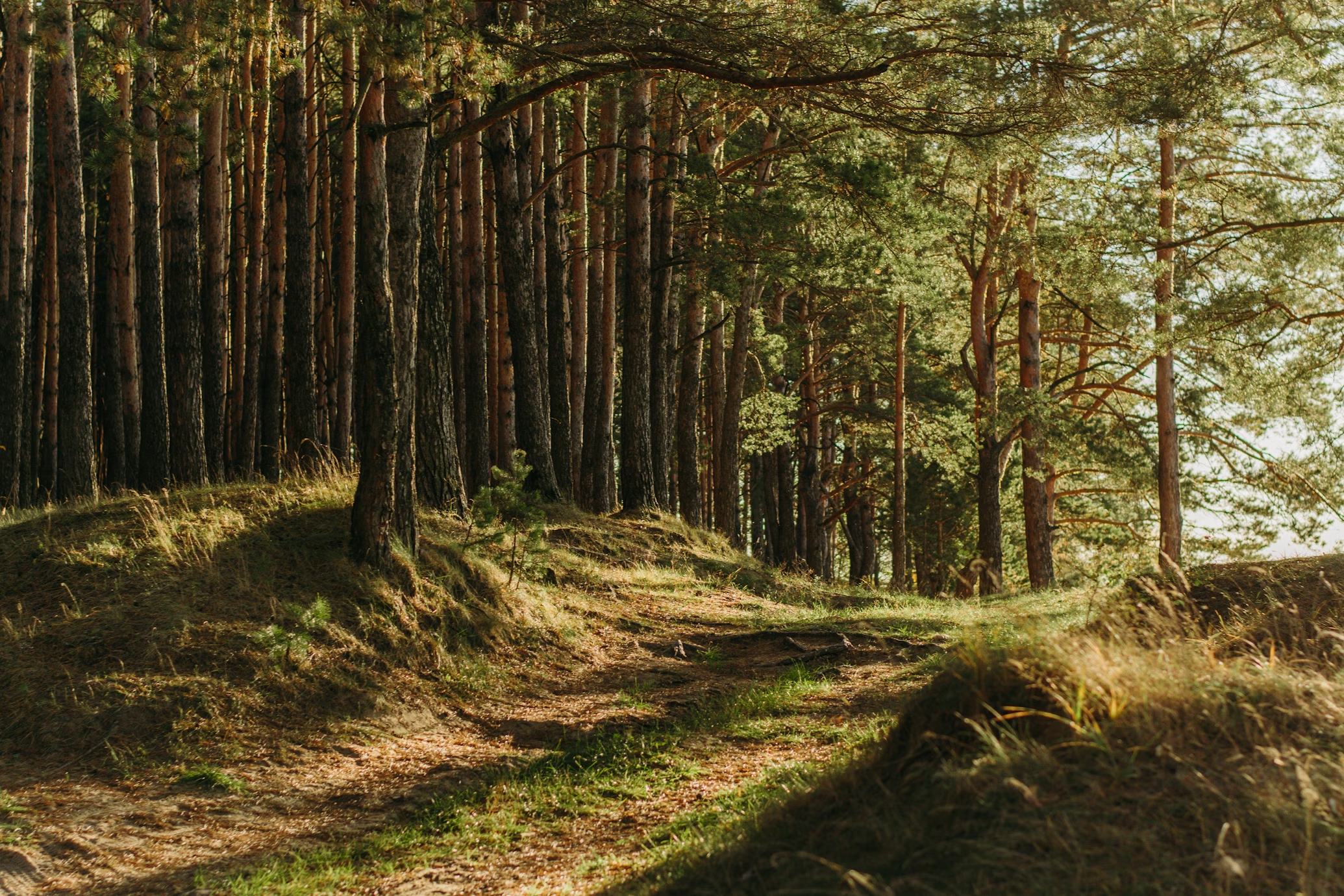
Monitoring of forest fuels (biomass, water content) in the boreal forest by multi-source satellite remote sensing
Context:
Nordic regions are warming more than twice as fast as the rest of the Globe with a significant impact on boreal forests. These ecosystems are subject to extremes events (droughts, fires) that become more frequent in the context of climate change. As a consequence, the role of boreal forests in the carbon budget can switch from carbon sinks to carbon sources. The increasing drying of boreal forests due to climate change, brings favorable vegetation and/or soil conditions for the outbreak of fires. As an example, the summer 2023 was particularly significant, as about 14.6 million hectares of Canadian forests burnt, impacting the ecosystems, wildlife habitat, air quality and the carbon budget. These boreal regions being vast and difficult to access, spatial remote sensing gives access to regular observations for monitoring forests of these northern regions. The objectives of the thesis are to take advantage of different satellite dataset to study key characteristics linked to plant fuels (biomass, water content of vegetation and soil) in boreal and Arctic regions, and their evolution related to extreme events (large fires and droughts) in a context of climate change.
To do so, it is planned to use data from several space missions, with a focus in the microwave spectral domain, such as the SMOS mission (Soil Moisture and Ocean Salinity-ESA/CNES), SMAP (Soil Moisture Active Passive-NASA), Sentinel-1 (ESA), ALOS PALSAR (JAXA), and also observations acquired in the optical domain from the Sentinel-2 mission. The use of LiDAR data from satellites such as ICESAT-2 or GEDI sensors can further be considered. From these datasets, the student will develop different methods such as the optimization of existing algorithms, multi-sensor synergy, machine learning and/or deep learning.
SMOS (left) and SMAP (right) are two satellites with L-band radiometers, whose missions focus on the water cycle with applications to vegetation. Credits: ESA/AOES Medialab, NASA/JPL-Caltech
In a first part, the study will focus on studying the main geophysical parameters (soil moisture, temperature, snow) which drive the evolution of biomass and water cycles in these northern regions and therefore the state of forest fuels. For example, in boreal regions, soil moisture and biomass in winter with the presence of snow, have a significant impact on the development of vegetation in spring and summer, and its ability to cope with extreme events. To improve our knowledge, the student will fine tune SMOS retrieval algorithm to derive the biomass in winter, with the presence of snow. In a second part, long time series will be analyzed to link the characteristics of the water state of the soil to the water state of the vegetation. This point will improve our understanding of the boreal forest response to extreme events such as drought. It will be necessary to analyze existing time series of drought index and vegetation (biomass and water content of vegetation) obtained by passive microwave remote sensing, in synergy with time series from other sensors. Finally, a final part will be devoted to evaluating the contribution of multisource remote sensing data to better monitor the vegetation before and after these extreme events (fires, drought, Arctic Oscillation, North Atlantic Oscillation), and to assess the interest of using satellite data in comparison with existing danger indices (such as the Canadian Forest Fire Danger Rating System in Canada).
Post wildfires in the Quebec region. Credits: Remi Madelon
These analyzes will eventually be extended by using the future Trishna satellite mission scheduled for launch in 2025, which could provide an interesting complement to the results of this work, in particular on water stress on vegetation.
Specificity of the thesis:
This joint supervision thesis will be conducted between Cesbio (Toulouse, France) and UTQR (Trois-Rivières, Quebec, Canada) during 4 years. The student will spend half his/her time at UTQR and the other half at Cesbio. This thesis will lead to a double diploma: a French and a Canadian thesis diploma. On the French side, this thesis will be supervised by Marie Parrens (Associate professor at INP Purpan) and Arnaud Mialon (HDR, Cesbio). On the Canadian side, it will be supervised by Alexandre Roy (Professor, UTQR) and Chelene Hanes (NR-Can).
Profile required:
Student with a 5-year degree in physics, geosciences or environmental sciences (university or engineering school) with an excellent academic record. Skills in Python or Matlab expected, with an interest in remote sensing and natural environments. Knowledge of machine learning/deep learning would be a plus. Student should be able to write scientific documents, including in English (French level required: C2; English level required: C1). Candidates must be self-motivated and able to work as part of a team.
How to apply:
For further information and to apply, please send a CV, covering letter and contact information for 2 references to the three future thesis supervisors:
– Marie Parrens : marie.parrens@purpan.fr
– Arnaud Mialon : arnaud.mialon@univ-tlse3.fr
– Alexandre Roy : alexandre.roy@uqtr.ca